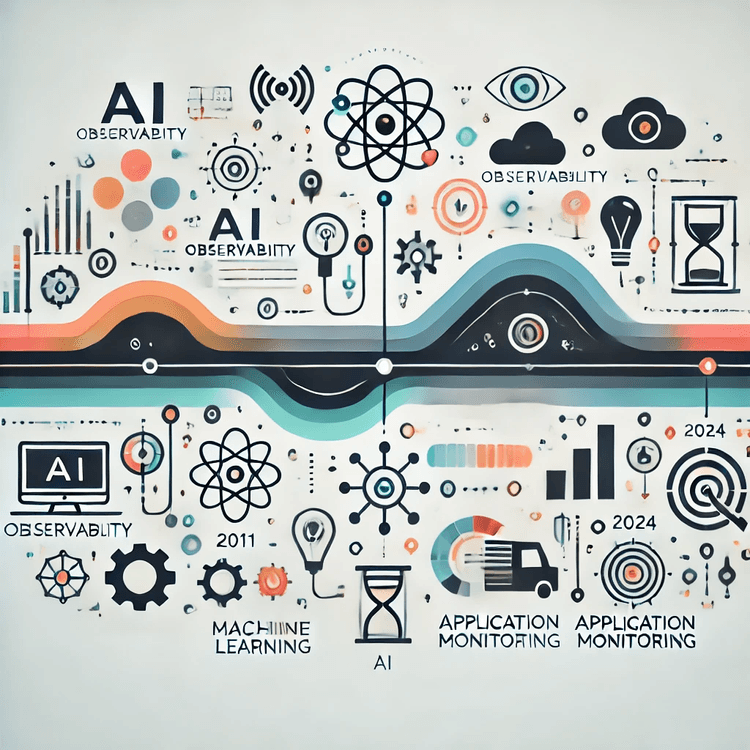
Evolving from ELK to AI-Powered Insights: Elastic’s Cutting-Edge Innovations
Discover the evolution of observability, from traditional monitoring to AI-powered insights. Learn how Elastic’s innovations like unified visibility, actionable insights, and machine learning are transforming IT operations for modern businesses.
Elastic, renowned for its ELK stack (Elasticsearch, Logstash, and Kibana), has established itself as a leader in the search, observability, and data analytics space over the last decade. The ELK stack, which started as a powerful trio for ingesting, searching, and visualizing data, laid the foundation for Elastic’s meteoric rise. Organizations from startups to enterprises quickly adopted the stack to gain meaningful insights from their log and metrics data. As data-driven decision-making became central to IT operations, Elastic evolved beyond the traditional ELK stack, introducing new capabilities that cater to the demands of modern observability and artificial intelligence. This roadmap of Elastic’s evolution tells the story of a company pushing boundaries while enabling businesses to achieve real-time operational excellence.
Elastic’s Proven Track Record of Innovation:
2011-2012:
• Introduction of the ELK stack (Elasticsearch, Logstash, Kibana)
• Initial focus on search and data visualization
2015-2017:
• Acquisition of Prelert to integrate machine learning
• Features introduced: anomaly detection and infrastructure monitoring
• Expansion into APM (Application Performance Monitoring)
2018:
• Acquisition of Opbeat for deeper APM capabilities
• Introduction of Index Lifecycle Management (ILM) to optimize data storage and retention
2019:
• Focus on AI/ML with features like:
• Anomaly detection
• Supervised learning
• Vector search support
• Adoption of W3C TraceContext for enhanced observability standards
2020:
• AI-powered detection and OpenTelemetry support
• Expansion to real user monitoring (RUM) and uptime tracking
• Introduction of OSQuery Manager for security insights
2021:
• Advancements in NLP (Natural Language Processing) and ML-based alerting
• Launch of Elastic Fleet and Agent for simplified data ingestion
• Searchable snapshots for cost-effective data management
2022:
Innovations like:
• Latency correlations
• Log categorization
• Cross-cluster search and replication
• APM for AWS Lambda
2023:
• Introduction of AI Assistant for proactive troubleshooting
• Log spike analysis and SLO monitoring tools
• Enhancements in Universal Profiling and Cloud Synthetics
2024 and Beyond:
New features include:
• ES|QL (query language for logs and metrics)
• RCA (Root Cause Analysis)
• Machine learning and generative AI advancements
• A vision of proactive, AI-powered observability
The Early Days: ELK and Beyond
The introduction of the ELK stack in 2011 marked a significant milestone in how companies managed their log data. Elasticsearch became the heart of this ecosystem, offering a lightning-fast, scalable search and analytics engine that could index data across massive volumes. Logstash and Kibana complemented Elasticsearch by providing powerful data ingestion and real-time visualization capabilities.
Together, they transformed log management into a cohesive and intuitive process for IT teams. Over the years, the ELK stack expanded its influence, particularly in the DevOps and IT operations spaces, where observability became a critical need. However, Elastic didn’t stop at just logs. It saw the need to address broader challenges, like correlating metrics, traces, and logs, which paved the way for a more unified observability solution.
Machine Learning and Anomaly Detection
Between 2015 and 2017, Elastic began its journey into machine learning and predictive analytics. The acquisition of Prelert, a pioneer in ML-powered anomaly detection, introduced a powerful new dimension to the Elastic stack. This allowed teams to proactively monitor for unusual behaviors, such as unexpected spikes in CPU usage or sudden drops in network traffic, even in massive datasets. By embedding machine learning directly into Elasticsearch, Elastic enabled teams to detect complex patterns and outliers without needing extensive data science expertise. These innovations helped IT teams transition from reactive troubleshooting to proactive incident prevention. As businesses grappled with increasing system complexity, these machine learning capabilities became essential for identifying root causes and mitigating risks before they impacted end users.
Expanding Observability
Elastic’s expansion into observability took a significant leap forward in 2018 with its acquisition of Opbeat, an application performance monitoring (APM) solution. This acquisition allowed Elastic to integrate application-level insights into its ecosystem, enabling teams to monitor, troubleshoot, and optimize application performance alongside infrastructure health. By combining APM with existing logging and metrics data, Elastic created a holistic observability platform that catered to modern development practices like microservices and distributed architectures.
During this time, the introduction of Index Lifecycle Management (ILM) further empowered users by providing tools to optimize data retention and storage. These advancements allowed Elastic to address common pain points like managing time-series data, ensuring compliance, and balancing cost-efficiency with performance.
AI and OpenTelemetry Integration
By 2019 and 2020, Elastic had firmly established itself as a leader in observability and data analytics, but the company wasn’t content to rest on its laurels. Instead, it turned its attention to the rapidly growing fields of AI and OpenTelemetry. With the rise of AIOps (AI for IT operations), Elastic introduced AI-powered anomaly detection, supervised learning models, and native support for vector search within Elasticsearch. These capabilities enabled more advanced insights, such as identifying patterns in high-cardinality data or understanding relationships across seemingly unrelated events. Additionally, Elastic’s adoption of OpenTelemetry, an open-source observability standard, demonstrated its commitment to interoperability. By supporting OpenTelemetry, Elastic ensured that organizations could easily integrate data from a wide range of systems, simplifying implementation and reducing the barriers to entry for observability.
Modern Advancements: NLP and AI Assistants
The years 2021 and beyond marked an inflection point in Elastic’s innovation, as it began incorporating more advanced AI and natural language processing (NLP) features into its platform. Latency correlations, log categorization, and automated root cause analysis (RCA) became key tools for simplifying complex troubleshooting workflows. By leveraging NLP, Elastic allowed users to ask natural-language questions about their data, making it easier for less technical teams to uncover actionable insights.
This user-centric approach was further enhanced by the introduction of AI assistants that could guide users through the platform, suggest optimizations, and automate repetitive tasks. These advancements reflect Elastic’s focus on democratizing observability and enabling organizations to extract maximum value from their data without needing deep technical expertise.
2024 and Beyond: ES|QL and Next-Generation Observability
Elastic’s 2024 roadmap highlights its vision for the future, with innovations like ES|QL (a powerful query language for logs and metrics) and universal profiling taking center stage. ES|QL simplifies how teams interact with observability data by offering a SQL-like syntax that is familiar to many IT professionals. This reduces the learning curve and empowers teams to query and analyze their data faster. Similarly, universal profiling allows organizations to gain end-to-end visibility into system performance, identifying bottlenecks across applications, infrastructure, and user interactions. As Elastic continues to innovate, it is helping organizations move beyond traditional observability practices to embrace AI-powered, proactive strategies that drive efficiency, improve reliability, and reduce costs.
Why Elastic Matters Today
Elastic’s commitment to innovation and its ability to anticipate the needs of modern organizations make it an invaluable partner in today’s data-driven world. By integrating features like anomaly detection, ML-based alerting, and OpenTelemetry support, Elastic enables organizations to monitor and optimize complex systems with ease. Its focus on AI-powered insights ensures that even non-technical users can extract meaningful information from their data. Whether you’re looking to improve Mean Time to Resolution (MTTR), enhance reliability, or optimize costs, Elastic’s observability platform provides the tools and flexibility to achieve your goals. With each new feature and enhancement, Elastic reaffirms its position as a leader in observability and a driving force behind operational excellence.
How O11yAI Can Help
At O11yAI, we specialize in helping organizations maximize the value of Elastic’s observability tools. Whether you’re looking to implement Elastic for the first time or optimize an existing deployment, our team of experts can guide you every step of the way. From setting up AI-powered anomaly detection to leveraging ES|QL for advanced querying, we ensure that your observability strategy delivers tangible business outcomes.
As Elastic continues to innovate, we stay ahead of the curve to help you make the most of these cutting-edge capabilities. Get in touch with us today to learn how we can transform your approach to observability.