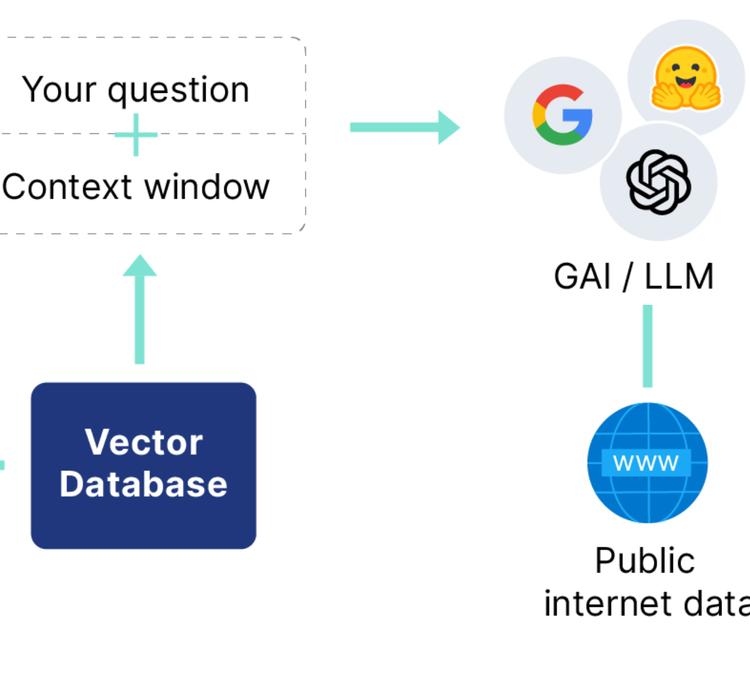
Top 10 Platforms for Building Retrieval-Augmented Generation (RAG) Systems in 2025
Discover the top 10 platforms for Retrieval-Augmented Generation (RAG) in 2025, including Elastic Enterprise Search, Pinecone, and more. Learn how these tools power AI with real-time data retrieval.
Introduction
The rise of Retrieval-Augmented Generation (RAG) revolutionized how AI models access and use external data to provide more accurate, relevant responses. For more on understanding how RAG systems enhance AI capabilities, explore our in-depth guide.
From customer support chatbots to enterprise knowledge bases, RAG workflows are becoming the cornerstone of cutting-edge AI applications. But to unlock the full potential of RAG, selecting the right platform is crucial.
In this blog, we’ll explore the top 10 platforms for RAG in 2025, showcasing industry leaders like Elastic Enterprise Search and its proven expertise (“You Know, for Search”) alongside innovative solutions like Pinecone, Weaviate, and more.
What is Retrieval-Augmented Generation (RAG)?
Retrieval-Augmented Generation bridges the gap between static AI knowledge and real-time data. It enhances LLM outputs by retrieving relevant external information before generating a response, ensuring:
• Accuracy: Up-to-date and factually correct answers.
• Efficiency: More cost-effective by pairing smaller LLMs with powerful retrieval systems.
• Scalability: Easily applied to diverse industries and workloads.
How RAG Works:
1. Retrieval Layer: Fetches relevant documents or data points from a database or index.
2. Generative Layer: Uses the retrieved data to craft precise, informed responses.
Top 10 Platforms for RAG in 2025
1. Elastic Enterprise Search
Elastic Enterprise Search is powered by Elasticsearch, the technology trusted by companies like Netflix, eBay, and Wikipedia. With over a decade of innovation, Elastic’s tagline “You Know, for Search” underscores its leadership in scalable, high-performance search and analytics.
Key Features:
• Hybrid Search: Combines keyword and vector-based semantic search for precise, relevant results.
• Real-Time Data Retrieval: Live indexing ensures responses are always based on the most current data.
• Enterprise-Grade Security: Protect sensitive data with role-based access control and encryption.
• LangChain Integration: Enables sophisticated RAG pipelines by seamlessly connecting Elastic with LLM orchestration frameworks.
Use Cases:
• Real-time product recommendations for e-commerce platforms.
• AI-powered customer support systems delivering accurate answers.
• Enterprise knowledge bases enabling teams to access information faster.
Why Elastic Stands Out:
Elastic’s proven expertise in search and its ability to scale reliably make it the go-to choice for organizations building RAG workflows. Whether you’re enhancing customer experiences or streamlining internal operations, Elastic delivers results you can trust.
Transform your RAG workflows with Elastic Enterprise Search. Learn more here.
2. Pinecone
Pinecone is a fully managed vector database designed for high-performance similarity searches, making it a natural fit for RAG systems.
Key Features:
• Scalable Infrastructure: Built for production-ready systems with high availability.
• Automatic Vector Management: Simplifies embedding storage and retrieval.
• Contextual Memory: Helps LLMs retain context for ongoing interactions.
Use Cases:
• Conversational agents with context-aware capabilities.
• AI-powered recommendation engines.
3. Weaviate
Weaviate is an open-source vector search engine offering flexibility, robust integrations, and scalability for diverse RAG applications.
Key Features:
• Hybrid Search: Merges keyword and semantic search capabilities.
• Pre-Built Integrations: Supports Hugging Face and OpenAI embeddings.
• GraphQL API: Advanced querying across structured and unstructured data.
Use Cases:
• Enterprise knowledge management systems.
• Semantic search for large datasets.
4. Milvus
Milvus is a high-performance vector database optimized for large-scale similarity search and machine learning applications.
Key Features:
• Multimodal Support: Handles text, image, and audio data.
• Kubernetes Integration: Scales effortlessly in distributed environments.
Use Cases:
• Video content retrieval systems.
• AI-driven analytics platforms.
5. Redis (RedisAI + Vector Search)
Redis combines its in-memory speed with vector search, delivering real-time performance for RAG workflows.
Key Features:
• Low Latency: Ideal for time-sensitive applications.
• AI Model Integration: Supports embeddings and similarity search.
Use Cases:
• Fraud detection systems.
• Personalized content recommendations.
6. LangChain
LangChain orchestrates RAG workflows by connecting LLMs with retrieval tools like Elastic or Pinecone.
Key Features:
• Custom Chains: Enables advanced, multi-step workflows.
• Prompt Optimization: Fine-tunes how LLMs interact with retrieved data.
Use Cases:
• AI-powered research tools.
• Document summarization systems.
7. Vespa
Vespa is an open-source platform for real-time hybrid search and machine learning-powered relevance ranking.
Key Features:
• Real-Time Indexing: Keeps search results current.
• Advanced Ranking: Customizable ranking algorithms for precision.
Use Cases:
• Media personalization platforms.
• Real-time content delivery systems.
8. Chroma
Chroma is a lightweight vector database, perfect for developers exploring small-scale RAG workflows.
Key Features:
• Ease of Use: Rapid setup for prototypes.
• Developer-Friendly: Minimal infrastructure overhead.
Use Cases:
• Prototyping semantic search tools.
• Small AI-driven applications.
9. OpenAI Embeddings API
OpenAI offers high-quality embeddings that integrate with RAG tools like Elastic or Pinecone.
Key Features:
• Scalable API: Simple to integrate with existing workflows.
• Versatile Embeddings: Suited for text, code, and more.
Use Cases:
• Document categorization systems.
• Semantic search workflows.
10. Haystack (deepset AI)
Haystack is an open-source RAG framework with pre-trained models and customizable pipelines.
Key Features:
• Pre-Trained Models: Speeds up development.
• Customizable Pipelines: Tailor workflows for specific needs.
Use Cases:
• AI-driven document retrieval.
• Enterprise knowledge management systems.
Conclusion
Elastic stands out as a trusted leader in search, offering unparalleled expertise and scalability. Whether you’re building AI chatbots or enterprise search solutions, Elastic’s “You Know, for Search” foundation makes it an ideal choice.
To ensure these platforms perform at their best, it’s important to leverage tools that provide transparency and efficiency. AI-driven observability plays a critical role in monitoring and enhancing the performance of RAG systems.
With so many powerful tools available, selecting and implementing the right RAG platform can be complex. Let us help you navigate the landscape, build scalable solutions, and achieve your AI goals. Talk to an expert to Get Started.